Artificial Intelligence (AI) has evolved significantly in recent decades, powering everything from personalized online recommendations to self-driving cars. As AI systems grow increasingly complex and diverse, it becomes crucial to manage and optimize these intelligent models holistically. Enter Meta Artificial Intelligence—the next frontier in AI research and development. Meta AI goes beyond traditional AI applications, focusing on creating intelligent systems capable of analyzing, designing, and improving other AI models. In this article, we will delve into the concept of Meta AI, its key components, real-world applications, and the challenges that lie ahead.
Table of Contents
What is Meta Artificial Intelligence?
Meta Artificial Intelligence, sometimes referred to as AI for AI, is a branch of artificial intelligence focused on building systems that can understand, evaluate, and optimize other AI models. Instead of solving a single task, Meta AI aims to improve the performance and efficiency of multiple AI or machine learning algorithms. By leveraging metadata—such as hyperparameters, architecture choices, and training processes—Meta AI seeks to create a more adaptive, self-improving ecosystem of intelligent applications.
How Meta AI Differs from Traditional AI
- Scope: Traditional AI is designed for a specific task—such as image recognition, natural language processing, or forecasting—while Meta AI looks at the broader architecture and process behind AI models.
- Self-Improvement: Unlike typical AI systems that rely on humans to tweak settings or design better architectures, Meta AI uses machine learning to automate these improvements.
- Adaptability: Because Meta AI evaluates multiple algorithms, it can intelligently switch or combine models based on changing data patterns or performance metrics.
Key Components of Meta AI
Meta AI relies on a combination of subfields and techniques that enable intelligent oversight, experimentation, and optimization of AI models. Some of the major components include:
Meta-Learning
Meta-learning, or learning to learn, is a technique where AI models are trained on a variety of tasks so that they can adapt quickly to new problems. This principle underpins Meta AI’s capability to evaluate and enhance existing AI systems. By understanding how different models learn, a meta-learning framework can derive insights and apply them to novel tasks or domains.
Automated Machine Learning (AutoML)
Automated Machine Learning (AutoML) tools automate the end-to-end process of deploying machine learning models. From feature selection to hyperparameter tuning, AutoML minimizes the human intervention needed in the AI development pipeline. Meta AI leverages AutoML to test different architectures efficiently and track performance metrics, ensuring quicker deployment without compromising on accuracy or scalability.
Reinforcement Learning and Control Systems
Reinforcement learning (RL) is a paradigm where an AI agent learns to make decisions by interacting with an environment and receiving rewards for favorable outcomes. In the context of Meta AI, RL can be used to make high-level decisions about AI model configurations—like selecting the right network architecture or training algorithm under different constraints. Meanwhile, control systems work in tandem with RL strategies to adjust learning rates, batch sizes, or other parameters in real time, steering AI models toward optimized performance.
Transfer Learning
Transfer learning allows AI models to use knowledge gained from one task to improve performance on another, related task. Meta AI employs transfer learning to rapidly adapt existing solutions or reuse certain modules instead of starting every project from scratch. This approach accelerates both training processes and model deployment, saving time and computational resources.
Applications and Impact
Meta AI stands to revolutionize the landscape of artificial intelligence by creating a more intelligent and self-sustaining ecosystem. Here are some key application areas:
Efficient Model Selection
Choosing the best AI model for a given problem can be time-consuming. Meta AI can analyze performance metrics, resource usage, and data size to recommend the most suitable model automatically. This approach saves valuable time and computational resources, allowing AI researchers and data scientists to focus on higher-level tasks, like interpreting results or refining business objectives.
Personalized AI Solutions
Meta AI’s adaptability makes it ideal for creating personalized experiences. By constantly monitoring user interactions and preferences, a Meta AI system can switch between various machine learning models or integrate new data to deliver tailored solutions. Whether it’s personalized content recommendations or dynamic pricing strategies, Meta AI ensures solutions remain up-to-date and relevant.
Robustness and Scalability
Large-scale AI deployments often face challenges related to model drift, data biases, and evolving data pipelines. Meta AI can monitor these parameters in real time, automatically identifying points of failure or inefficiencies. Once identified, it can either correct these issues or switch to alternative models. This proactive approach ensures stable and robust solutions, even as data or business conditions change.
Cross-Domain Innovation
Meta AI’s ability to analyze and optimize AI models across multiple fields encourages cross-domain innovation. For instance, insights gained from optimizing image recognition systems could inform natural language processing models about efficient architectural patterns or training routines. This cross-pollination of knowledge accelerates breakthroughs and drives the AI field forward.
Challenges and Considerations
While Meta AI is poised to transform how we build and maintain AI systems, it also comes with its own set of complexities and challenges:
Data Complexity
Meta AI often needs access to detailed metadata about model architectures, hyperparameters, and training processes. In many organizations, this metadata is either siloed across teams or not collected in a standardized format. Consolidating such vast and varied data can be a significant obstacle when deploying Meta AI at scale.
Computation and Resource Management
Training AI models is computationally expensive—and training multiple AI models to optimize themselves can push resource requirements even higher. Without efficient strategies for parallelization and resource management, the cost and time needed to train a Meta AI system can be prohibitive for many organizations.
Interpretability and Explainability
Just as with advanced deep learning models, Meta AI systems can be complex, making their decision-making processes difficult to interpret. Organizations may face regulatory or ethical requirements for explainability, especially in sensitive sectors like healthcare or finance. Balancing complexity with the need for transparency is an ongoing challenge.
Ethical and Security Concerns
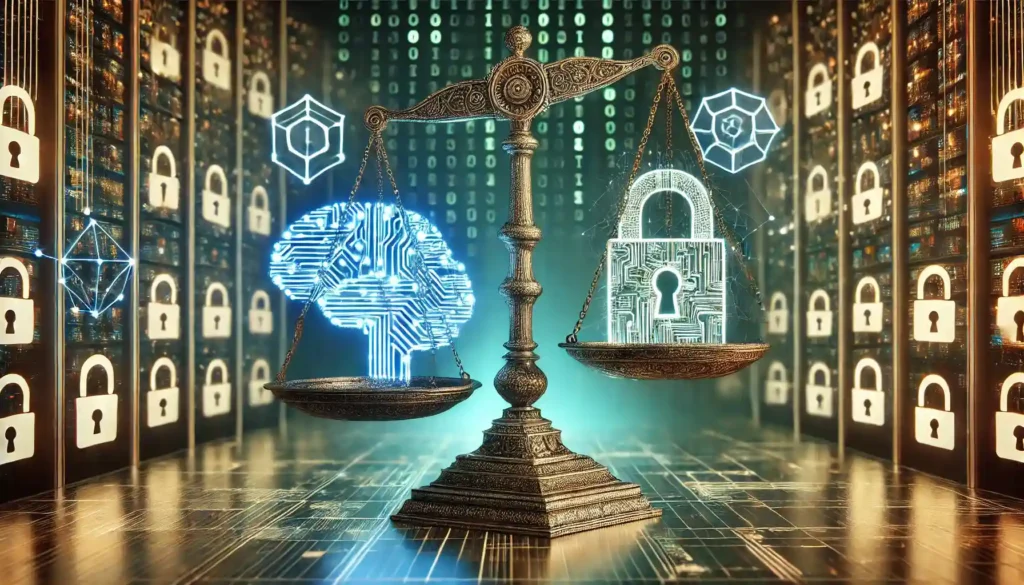
Meta AI’s power to oversee and optimize other AI algorithms also raises ethical questions about data privacy and security. If a malicious actor gains access to a Meta AI system, they could manipulate the entire AI ecosystem. Additionally, Meta AI models that lack proper guardrails may inadvertently optimize for outcomes that conflict with human values, highlighting the importance of human oversight and robust security protocols.
Best Practices for Implementing Meta AI
Implementing a Meta AI framework involves strategic planning, robust infrastructure, and a clear understanding of organizational objectives. Below are some best practices:
- Centralize Metadata: To effectively train Meta AI, consolidate metadata from various AI projects. This includes model configurations, performance metrics, training logs, and deployment details.
- Adopt Incremental Approaches: Instead of attempting to meta-optimize all AI projects simultaneously, start with a few high-impact models. Gradually expand the Meta AI’s responsibilities as it proves its effectiveness.
- Focus on Governance: Establish guidelines for data governance, model versioning, and accountability. This ensures that Meta AI has reliable, high-quality information on which to base its decisions.
- Invest in Infrastructure: Ensure your organization has sufficient computational resources and a flexible architecture to support scalable training and experimentation. A well-designed cloud or hybrid infrastructure often proves beneficial in this regard.
- Maintain Human in the Loop: While Meta AI automates many processes, retaining human oversight for ethical and interpretability checks is essential. This prevents harmful biases or unforeseen consequences from scaling across multiple AI systems.
Future Outlook
As AI applications become more ubiquitous, Meta AI will likely play a key role in orchestrating and optimizing these complex systems. Researchers are exploring meta-genetic algorithms and meta-reinforcement learning methods to further automate the creation of model architectures. In addition, advancements in distributed computing and quantum computing could enable Meta AI to handle even larger search spaces, making optimization both faster and more powerful.
The impact of Meta AI could extend beyond enterprise applications. Healthcare, for instance, could benefit from more personalized and adaptable diagnostic models, while industries like energy management could see AI-driven optimizations that adapt swiftly to real-time demand and supply fluctuations. In essence, Meta AI holds the potential to reshape the very fabric of AI research, turning it from an endeavor driven by human intuition and trial-and-error to a systematic, data-driven process guided by advanced meta-systems.
Conclusion
Meta Artificial Intelligence represents a new paradigm in how we develop, deploy, and refine AI applications. By creating self-improving systems that leverage metadata for optimization, organizations can scale AI solutions more efficiently and deliver personalized, robust, and adaptive models. Despite the challenges—ranging from computational costs to ethical considerations—Meta AI’s promise is significant. As research continues and more organizations recognize its potential, Meta AI will likely become an essential component of any cutting-edge AI strategy.
For further reading on
meta-learning and advanced AI strategies, you can explore this resource from MIT Technology Review.
open AI technologie